What is Deep Learning?
The Revolutionary Role of Deep Learning in Cybersecurity and Antivirus: Exploring the Advanced Capabilities of Neural Network Methodology
Deep learning is a subfield of
machine learning and
artificial intelligence that has evolved in recent years, transforming the field of cybersecurity and
antivirus. Deep learning is a complex layered neural network methodology that uses large-scale data processing to build sophisticated computational models. Deep learning models are capable of learning complex mappings between inputs and outputs, providing a means of performing intricate tasks of
pattern recognition,
natural language processing, speech, and
image analysis by automatically discovering features in the raw data sets.
deep learning is being used to detect and prevent sophisticated malware, including
zero-day threats,
advanced persistent threats (APTs), and trojans. Traditional antivirus systems are signature-based and can only detect known malware threats. As an increasing number of
cyber threats have continued to emerge, with different variations and combinations, attackers have adopted tactics that bypass signature-based defenses by using advanced techniques to transform malware payload (e.g., encryption) and conceal them.
Deep learning based
security solutions, on the other hand, leverage a range of automated and intelligent features that use
heuristic analysis, clustering, and classification algorithms to detect and stop previously undiscovered cyber-attacks. Deep learning can classify, identify and even predict the behavior of malware with exponentially greater precision, efficiency, and speed. According to a report by the International Data Corp, these capabilities outperform conventional
malware detection methods significantly.
Deep learning-based security solutions use large amounts of data to create a deep-layered neural network that then permits the machine learning algorithm to identify previously undiscovered patterns and variables to automatically classify the new findings. These deep learning models build on supervised or unsupervised methods, and they learn directly from the data without the need for manual labeling. Deep learning allows for non-linear relationships between data sets to be modeled, which can recognize often complicating and hidden properties in raw data and see patterns otherwise unseen by the human eye.
What differentiates deep learning-based security solutions is the approach taken to prevent and detect malware. Deep learning-based platforms compared to the signature and heuristic security remedies push the detection continuum closer to detecting cross-opting malware (evasion tactics changing regularly victimization). Deep learning models can help antivirus programs automatically optimize as more malware emerges.
Deep learning also “promises to shift the risk landscape,” promoting prevention, awareness, and resilience to malware ahead of victimizing targets by identifying vulnerabilities, removing susceptibility levels, so exploitation can cease beforehand or at the outset. The results are better-scoring outcomes for individual workplaces, sectors, and industries.
One important avenue that drives growth in deep learning-initiated cybersecurity is IoT (Internet of Things). IoT networks mainly operate machine-to-machine without involving humans. This interchange is attractive to bots (programs that infect a device locally or over a shared network) since all weaknesses become gateway opportunities into a connected network, meaning machines communicating with other machines getting hit with malware. Deep learning based security solutions improve the efficiency of
threat detection using advanced techniques, such as dense network information extraction and NLP (Natural Language Processing machinery). The IoT aegis platform consists of sensors monitoring devices in 330 locations. Adapting is necessary, as the need to scrutinize arrangements change. Deep learning leads to calculated solutions by adapting protocols augmenting cybersecurity market preventive efforts.
Although the use of Deep learning in antivirus is a considerable milestone for the industry, continuous scrutiny is necessary. The overwhelming power deep learning possesses constitutes a double-edged sword that potential attackers can very well wield. The capacity of deep learning to gain incredible accuracy delivered at speeds unnervingly quicker than the human reflex, opens vulnerabilities of the classification choice by the AI. Hence, the software will be tactically flawed so that attackers can evade the protections.
Security knowledge is in continuous mode—it begs to be replicated often as new attackers, their processes, trojans, and viruses crop entering fortresses put up by developers to mitigate. Building and maintaining new intruder practices discernment models are vital to understanding the attackers’ psyche finally. Notably, in cybersecurity machine-oriented supervision invades human-centered
risk management frameworks. With that necessity, mapping deep learning’s
best practices regulate precautionary controls that subvert tropes typical in IP (information protected) theft scenarios happen fast—witness massive breaches occurring in most sized businesses. From healthcare sites to larger scale shops storing personnel data closing hastily due to lack of
surveillance behind digital wallet seal.
deep learning has exceptional potential when it comes to cybersecurity and antivirus, being proficient at handling vast amounts of complex and nuanced data without significant loss of accuracy, and could serve specific interests in encryption, low-latency detection (that identifies infected files in hidden areas), natural language processing, vehicular devices, and industrial controls. better deep learning initiatives that encapsulate generated test evaluation interest are not constrained or played with through rules fixed from traditional programmed malware detectors, endorsing rapid, and live, self-developing systems that integrate into an individual customized manner typical in cybersecurity requirements. to be successful in implementing machine-operated systems coupled to automation (quantum computing) software paradigm shifts prepare developers during a massive shift adapted to networks thought impossible delivering as promising indicators for more profound implementation soon representing increased security to corporations with AI tracking sustainability metrics on deviation output expected.
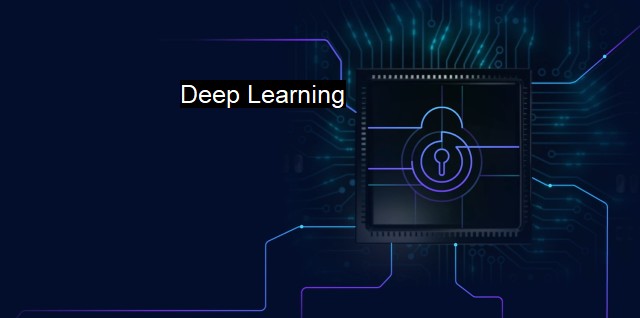
Deep Learning FAQs
What is deep learning and how does it relate to cybersecurity?
Deep learning is a subset of machine learning that involves training artificial neural networks to recognize patterns and features in data. In cybersecurity, deep learning can be used for tasks such as identifying and detecting malware and other threats.How does deep learning improve antivirus software performance?
Deep learning allows antivirus software to learn and adapt to new threats in real-time, improving its ability to detect and prevent malware. By analyzing patterns in large datasets and identifying similarities between known threats and new malware, deep learning algorithms can improve the accuracy and speed of antivirus software.What are some challenges with implementing deep learning in cybersecurity?
One challenge is the need for high-quality data to train deep learning algorithms. This requires collecting and labeling large datasets of malware and other threats, which can be time-consuming and resource-intensive. Another challenge is the potential for adversaries to use deep learning to evade detection by antivirus software, which requires constant updates and improvements to stay ahead of emerging threats.How can deep learning be used to enhance network security?
Deep learning can be used to monitor network traffic and identify anomalies or suspicious behavior that may indicate a cyber attack. This can include detecting unusual patterns of data transfer or identifying attempts to access restricted areas of the network. Deep learning algorithms can also be used for intrusion detection and prevention, flagging potential threats and taking action to mitigate them before they cause damage.